Synthetic organic chemistry driven by artificial intelligence
Synthetic organic chemistry underpins several areas of chemistry, including drug discovery, chemical biology, materials science and engineering. However, the execution of complex chemical syntheses in itself requires expert knowledge, usually acquired over many years of study and hands-on laboratory practice. The development of technologies with potential to streamline and automate chemical synthesis is a half-century-old endeavour yet to be fulfilled. Renewed interest in artificial intelligence (AI), driven by improved computing power, data availability and algorithms, is overturning the limited success previously obtained. In this Review, we discuss the recent impact of AI on different tasks of synthetic chemistry and dissect selected examples from the literature. By examining the underlying concepts, we aim to demystify AI for bench chemists in order that they may embrace it as a tool rather than fear it as a competitor, spur future research by pinpointing the gaps in knowledge and delineate how chemical AI will run in the era of digital chemistry.
This is a preview of subscription content, access via your institution
Access options
Access Nature and 54 other Nature Portfolio journals
Get Nature+, our best-value online-access subscription
cancel any time
Subscribe to this journal
Receive 12 digital issues and online access to articles
133,45 € per year
only 11,12 € per issue
Buy this article
- Purchase on SpringerLink
- Instant access to full article PDF
Prices may be subject to local taxes which are calculated during checkout
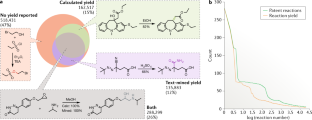
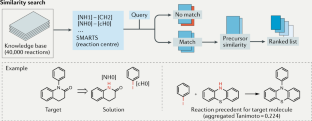
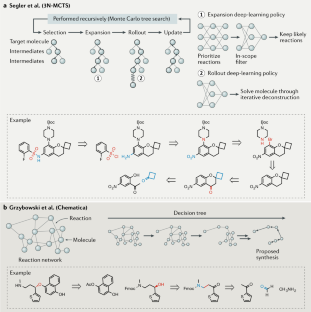
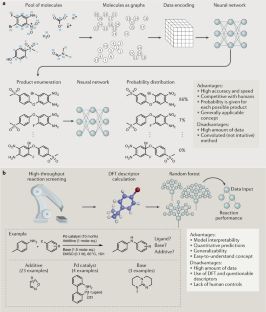
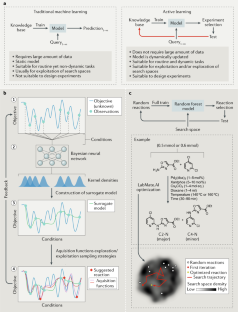
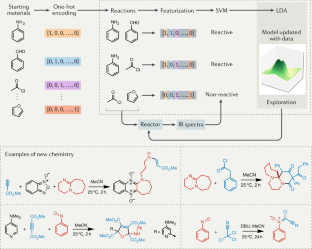
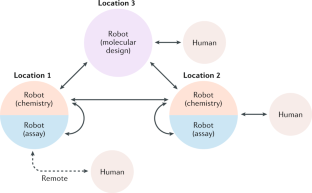
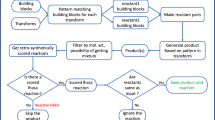
SAVI, in silico generation of billions of easily synthesizable compounds through expert-system type rules
Article Open access 11 November 2020
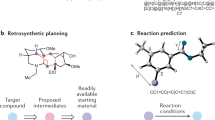
Automation and computer-assisted planning for chemical synthesis
Article 18 March 2021
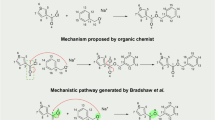
A large-scale reaction dataset of mechanistic pathways of organic reactions
Article Open access 10 August 2024
References
- Nantermet, P. G. Reaction: the art of synthetic chemistry. Chem1, 335–336 (2016). CASGoogle Scholar
- Nicolaou, K. C. & Chen, J. S. The art of total synthesis through cascade reactions. Chem. Soc. Rev.38, 2993–3009 (2009). CASPubMedPubMed CentralGoogle Scholar
- Baran, P. S. Natural product total synthesis: as exciting as ever and here to stay. J. Am. Chem. Soc.140, 4751–4755 (2018). CASPubMedGoogle Scholar
- Ley, S. V. The engineering of chemical synthesis: humans and machines working in harmony. Angew. Chem. Int. Ed.57, 5182–5183 (2018). CASGoogle Scholar
- Bergman, R. G. & Danheiser, R. L. Reproducibility in chemical research. Angew. Chem. Int. Ed.55, 12548–12549 (2016). CASGoogle Scholar
- Duros, V. et al. Human versus robots in the discovery and crystallization of gigantic polyoxometalates. Angew. Chem. Int. Ed.56, 10815–10820 (2017). CASGoogle Scholar
- Roch, L. M. et al. ChemOS: Orchestrating autonomous experimentation. Science Robot.3, eaat5559 (2018). Google Scholar
- Schneider, G. Mind and machine in drug design. Nat. Mach. Intell.1, 128–130 (2019). Google Scholar
- Wang, Y. et al. Acoustic droplet ejection enabled automated reaction scouting. ACS Cent. Sci.5, 451–457 (2019). CASPubMedPubMed CentralGoogle Scholar
- Fitzpatrick, D. E., Battilocchio, C. & Ley, S. V. Enabling technologies for the future of chemical synthesis. ACS Cent. Sci.2, 131–138 (2016). CASPubMedPubMed CentralGoogle Scholar
- Ley, S. V., Fitzpatrick, D. E., Myers, R. M., Battilocchio, C. & Ingham, R. J. Machine-assisted organic synthesis. Angew. Chem. Int. Ed.54, 10122–10136 (2015). CASGoogle Scholar
- Lehmann, J. W., Blair, D. J. & Burke, M. D. Toward generalization of iterative small molecule synthesis. Nat. Rev. Chem.2, 0115 (2018). PubMedPubMed CentralGoogle Scholar
- Corey, E. J. & Wipke, W. T. Computer-assisted design of complex organic syntheses. Science166, 178–192 (1969). CASPubMedGoogle Scholar
- Pensak, D. A. & Corey, E. J. in Computer-Assisted Organic Synthesis Ch. 1 (eds Wipke, W. T. & Howe, W. J.) 1-32 (American Chemical Society, 1977).
- Lajiness, M. S., Maggiora, G. M. & Shanmugasundaram, V. Assessment of the consistency of medicinal chemists in reviewing sets of compounds. J. Med. Chem.47, 4891–4896 (2004). CASPubMedGoogle Scholar
- Earkin, D. R. & Warr, W. A. in Computer-Assisted Organic Synthesis Ch. 10 (eds Wipke, W. T. & Howe, W. J.) 217-226 (American Chemical Society, 1977).
- Sridharan, N. S. in Computer-Assisted Organic Synthesis Ch. 7 (eds Wipke, W. T. & Howe, W. J.) 148-178 (American Chemical Society, 1977).
- Wipke, W. T., Ouchi, G. I. & Krishnan, S. Simulation and evaluation of chemical synthesis—SECS: An application of artificial intelligence techniques. Artif. Intell.11, 173–193 (1978). Google Scholar
- Hessler, G. & Baringhaus, K. H. Artificial intelligence in drug design. Molecules23, E2520 (2018). PubMedGoogle Scholar
- Sellwood, M. A., Ahmed, M., Segler, M. H. & Brown, N. Artificial intelligence in drug discovery. Future Med. Chem.10, 2025–2028 (2018). CASPubMedGoogle Scholar
- Aspuru-Guzik, A., Lindh, R. & Reiher, M. The matter simulation (r)evolution. ACS Cent. Sci.4, 144–152 (2018). CASPubMedPubMed CentralGoogle Scholar
- Lusher, S. J., McGuire, R., van Schaik, R. C., Nicholson, C. D. & de Vlieg, J. Data-driven medicinal chemistry in the era of big data. Drug Discov. Today19, 859–868 (2014). CASPubMedGoogle Scholar
- Tetko, I. V., Engkvist, O., Koch, U., Reymond, J. L. & Chen, H. BIGCHEM: challenges and opportunities for big data analysis in chemistry. Mol. Inf.35, 615–621 (2016). CASGoogle Scholar
- Henson, A. B., Gromski, P. S. & Cronin, L. Designing algorithms to aid discovery by chemical robots. ACS Cent. Sci.4, 793–804 (2018). CASPubMedPubMed CentralGoogle Scholar
- Rich, A. S. & Gureckis, T. M. Lessons for artificial intelligence from the study of natural stupidity. Nat. Mach. Intell.1, 174–180 (2019). Google Scholar
- Ekins, S. et al. Exploiting machine learning for end-to-end drug discovery and development. Nat. Mater.18, 435–441 (2019). CASPubMedPubMed CentralGoogle Scholar
- Wishart, D. S. et al. DrugBank 5.0: a major update to the DrugBank database for 2018. Nucleic Acids Res.46, D1074–D1082 (2018). CASPubMedGoogle Scholar
- Gaulton, A. et al. The ChEMBL database in 2017. Nucleic Acids Res.45, D945–D954 (2017). CASPubMedGoogle Scholar
- Kim, S. et al. PubChem 2019 update: improved access to chemical data. Nucleic Acids Res.47, D1102–D1109 (2019). PubMedGoogle Scholar
- Grzybowski, B. A. et al. Chematica: A story of computer code that started to think like a chemist. Chem4, 390–398 (2018). CASGoogle Scholar
- Segler, M. H. S., Preuss, M. & Waller, M. P. Planning chemical syntheses with deep neural networks and symbolic AI. Nature555, 604–610 (2018). CASPubMedGoogle Scholar
- Schneider, N., Lowe, D. M., Sayle, R. A., Tarselli, M. A. & Landrum, G. A. Big data from pharmaceutical patents: A computational analysis of medicinal chemists’ bread and butter. J. Med. Chem.59, 4385–4402 (2016). CASPubMedGoogle Scholar
- Ahneman, D. T., Estrada, J. G., Lin, S., Dreher, S. D. & Doyle, A. G. Predicting reaction performance in C–N cross-coupling using machine learning. Science360, 186–190 (2018). CASPubMedGoogle Scholar
- Roughley, S. D. & Jordan, A. M. The medicinal chemist’s toolbox: an analysis of reactions used in the pursuit of drug candidates. J. Med. Chem.54, 3451–3479 (2011). CASPubMedGoogle Scholar
- Lowe, D. AI designs organic syntheses. Nature555, 592–593 (2018). CASPubMedGoogle Scholar
- Coley, C. W., Green, W. H. & Jensen, K. F. Machine learning in computer-aided synthesis planning. Acc. Chem. Res.51, 1281–1289 (2018). CASPubMedGoogle Scholar
- Gelernter, H. L. et al. Empirical explorations of SYNCHEM. Science197, 1041–1049 (1977). CASPubMedGoogle Scholar
- Cadeddu, A., Wylie, E. K., Jurczak, J., Wampler-Doty, M. & Grzybowski, B. A. Organic chemistry as a language and the implications of chemical linguistics for structural and retrosynthetic analyses. Angew. Chem. Int. Ed.53, 8108–8112 (2014). CASGoogle Scholar
- Coley, C. W., Rogers, L., Green, W. H. & Jensen, K. F. Computer-assisted retrosynthesis based on molecular similarity. ACS Cent. Sci.3, 1237–1245 (2017). CASPubMedPubMed CentralGoogle Scholar
- Hartenfeller, M. et al. DOGS: reaction-driven de novo design of bioactive compounds. PLoS Comput. Biol.8, e1002380 (2012). CASPubMedPubMed CentralGoogle Scholar
- Rodrigues, T. et al. De novo design and optimization of Aurora A kinase inhibitors. Chem. Sci.4, 1229–1233 (2013). CASGoogle Scholar
- Rodrigues, T. et al. Steering target selectivity and potency by fragment-based de novo drug design. Angew. Chem. Int. Ed.52, 10006–10009 (2013). CASGoogle Scholar
- Friedrich, L., Rodrigues, T., Neuhaus, C. S., Schneider, P. & Schneider, G. From complex natural products to simple synthetic mimetics by computational de novo design. Angew. Chem. Int. Ed.55, 6789–6792 (2016). CASGoogle Scholar
- Lewell, X. Q., Judd, D. B., Watson, S. P. & Hann, M. M. RECAP — retrosynthetic combinatorial analysis procedure: a powerful new technique for identifying privileged molecular fragments with useful applications in combinatorial chemistry. J. Chem. Inf. Comput. Sci.38, 511–522 (1998). CASPubMedGoogle Scholar
- Reker, D., Bernardes, G. J. L. & Rodrigues, T. Computational advances in combating colloidal aggregation in drug discovery. Nat. Chem.11, 402–418 (2019). CASPubMedGoogle Scholar
- Liu, B. et al. Retrosynthetic reaction prediction using neural sequence-to-sequence models. ACS Cent. Sci.3, 1103–1113 (2017). CASPubMedPubMed CentralGoogle Scholar
- Altae-Tran, H., Ramsundar, B., Pappu, A. S. & Pande, V. Low data drug discovery with one-shot learning. ACS Cent. Sci.3, 283–293 (2017). CASPubMedPubMed CentralGoogle Scholar
- Chen, H., Engkvist, O., Wang, Y., Olivecrona, M. & Blaschke, T. The rise of deep learning in drug discovery. Drug Discov. Today23, 1241–1250 (2018). PubMedGoogle Scholar
- Ching, T. et al. Opportunities and obstacles for deep learning in biology and medicine. J. R. Soc. Interface15, 20170387 (2018). PubMedPubMed CentralGoogle Scholar
- Baylon, J. L., Cilfone, N. A., Gulcher, J. R. & Chittenden, T. W. Enhancing retrosynthetic reaction prediction with deep learning using multiscale reaction classification. J. Chem. Inf. Model.59, 673–688 (2019). CASPubMedGoogle Scholar
- Fialkowski, M., Bishop, K. J., Chubukov, V. A., Campbell, C. J. & Grzybowski, B. A. Architecture and evolution of organic chemistry. Angew. Chem. Int. Ed.44, 7263–7269 (2005). CASGoogle Scholar
- Gothard, C. M. et al. Rewiring chemistry: algorithmic discovery and experimental validation of one-pot reactions in the network of organic chemistry. Angew. Chem. Int. Ed.51, 7922–7927 (2012). CASGoogle Scholar
- Grzybowski, B. A., Bishop, K. J., Kowalczyk, B. & Wilmer, C. E. The ‘wired’ universe of organic chemistry. Nat. Chem.1, 31–36 (2009). CASPubMedGoogle Scholar
- Kowalik, M. et al. Parallel optimization of synthetic pathways within the network of organic chemistry. Angew. Chem. Int. Ed.51, 7928–7932 (2012). CASGoogle Scholar
- Segler, M. H. S. & Waller, M. P. Neural-symbolic machine learning for retrosynthesis and reaction prediction. Chem. Eur. J.23, 5966–5971 (2017). CASPubMedGoogle Scholar
- Silver, D. et al. Mastering the game of Go with deep neural networks and tree search. Nature529, 484–489 (2016). CASPubMedGoogle Scholar
- Browne, C. et al. A survey of Monte Carlo tree search methods. IEEE Trans. Comput. Intell. AI Games4, 1–43 (2012). Google Scholar
- Schreck, J. S., Coley, C. W. & Bishop, K. J. M. Learning retrosynthetic planning through simulated experience. ACS Cent. Sci.5, 970–981 (2019). CASPubMedPubMed CentralGoogle Scholar
- Szymkuc, S. et al. Computer-assisted synthetic planning: The end of the beginning. Angew. Chem. Int. Ed.55, 5904–5937 (2016). CASGoogle Scholar
- Klucznik, T. et al. Efficient syntheses of diverse, medicinally relevant targets planned by computer and executed in the laboratory. Chem4, 522–532 (2018). CASGoogle Scholar
- Molga, K., Dittwald, P. & Grzybowski, B. A. Navigating around patented routes by preserving specific motifs along computer-planned retrosynthetic pathways. Chem5, 460–473 (2019). CASGoogle Scholar
- Badowski, T., Molga, K. & Grzybowski, B. A. Selection of cost-effective yet chemically diverse pathways from the networks of computer-generated retrosynthetic plans. Chem. Sci.10, 4640–4651 (2019). CASPubMedPubMed CentralGoogle Scholar
- Burke, K. Perspective on density functional theory. J. Chem. Phys.136, 150901 (2012). PubMedGoogle Scholar
- Chermette, H. Chemical reactivity indexes in density functional theory. J. Comput. Chem.20, 129–154 (1999). CASGoogle Scholar
- Hegde, G. & Bowen, R. C. Machine-learned approximations to density functional theory Hamiltonians. Sci. Rep.7, 42669 (2017). CASPubMedPubMed CentralGoogle Scholar
- Smith, J. S., Isayev, O. & Roitberg, A. E. ANI-1: an extensible neural network potential with DFT accuracy at force field computational cost. Chem. Sci.8, 3192–3203 (2017). CASPubMedPubMed CentralGoogle Scholar
- Grisafi, A. et al. Transferable machine-learning model of the electron density. ACS Cent. Sci.5, 57–64 (2019). CASPubMedGoogle Scholar
- Sadowski, P., Fooshee, D., Subrahmanya, N. & Baldi, P. Synergies between quantum mechanics and machine learning in reaction prediction. J. Chem. Inf. Model.56, 2125–2128 (2016). CASPubMedGoogle Scholar
- Moosavi, S. M. et al. Capturing chemical intuition in synthesis of metal-organic frameworks. Nat. Commun.10, 539 (2019). CASPubMedPubMed CentralGoogle Scholar
- Raccuglia, P. et al. Machine-learning-assisted materials discovery using failed experiments. Nature533, 73–76 (2016). CASPubMedGoogle Scholar
- Kayala, M. A., Azencott, C. A., Chen, J. H. & Baldi, P. Learning to predict chemical reactions. J. Chem. Inf. Model.51, 2209–2222 (2011). CASPubMedPubMed CentralGoogle Scholar
- Fooshee, D. et al. Deep learning for chemical reaction prediction. Mol. Syst. Des. Eng.3, 442–452 (2018). CASGoogle Scholar
- Schwaller, P., Gaudin, T., Lanyi, D., Bekas, C. & Laino, T. “Found in Translation”: predicting outcomes of complex organic chemistry reactions using neural sequence-to-sequence models. Chem. Sci.9, 6091–6098 (2018). CASPubMedPubMed CentralGoogle Scholar
- Wei, J. N., Duvenaud, D. & Aspuru-Guzik, A. Neural networks for the prediction of organic chemistry reactions. ACS Cent. Sci.2, 725–732 (2016). CASPubMedPubMed CentralGoogle Scholar
- Hughes, T. B., Dang, N. L., Miller, G. P. & Swamidass, S. J. Modeling reactivity to biological macromolecules with a deep multitask network. ACS Cent. Sci.2, 529–537 (2016). CASPubMedPubMed CentralGoogle Scholar
- Hughes, T. B., Miller, G. P. & Swamidass, S. J. Modeling epoxidation of drug-like molecules with a deep machine learning network. ACS Cent. Sci.1, 168–180 (2015). CASPubMedPubMed CentralGoogle Scholar
- Coley, C. W., Barzilay, R., Jaakkola, T. S., Green, W. H. & Jensen, K. F. Prediction of organic reaction outcomes using machine learning. ACS Cent. Sci.3, 434–443 (2017). CASPubMedPubMed CentralGoogle Scholar
- Coley, C. W. et al. A graph-convolutional neural network model for the prediction of chemical reactivity. Chem. Sci.10, 370–377 (2019). CASPubMedGoogle Scholar
- Breiman, L. Random forests. Mach. Learn.45, 5–32 (2001). Google Scholar
- Ho, T. K. The random subspace method for constructing decision forests. IEEE Trans. Pattern Anal. Mach. Intell.20, 832–844 (1998). Google Scholar
- Rodrigues, T. et al. De novo fragment design for drug discovery and chemical biology. Angew. Chem. Int. Ed.54, 15079–15083 (2015). CASGoogle Scholar
- Rodrigues, T. et al. Machine intelligence decrypts beta-lapachone as an allosteric 5-lipoxygenase inhibitor. Chem. Sci.9, 6899–6903 (2018). CASPubMedPubMed CentralGoogle Scholar
- Richter, M. F. et al. Predictive compound accumulation rules yield a broad-spectrum antibiotic. Nature545, 299–304 (2017). CASPubMedPubMed CentralGoogle Scholar
- Wolfe, J. M. et al. Machine learning to predict cell-penetrating peptides for antisense delivery. ACS Cent. Sci.4, 512–520 (2018). CASPubMedPubMed CentralGoogle Scholar
- Chuang, K. V. & Keiser, M. J. Comment on “Predicting reaction performance in C–N cross-coupling using machine learning”. Science362, eaat8603 (2018). PubMedGoogle Scholar
- Estrada, J. G., Ahneman, D. T., Sheridan, R. P., Dreher, S. D. & Doyle, A. G. Response to Comment on “Predicting reaction performance in C–N cross-coupling using machine learning”. Science362, eaat8763 (2018). PubMedGoogle Scholar
- Skoraczynski, G. et al. Predicting the outcomes of organic reactions via machine learning: are current descriptors sufficient? Sci. Rep.7, 3582 (2017). CASPubMedPubMed CentralGoogle Scholar
- Chuang, K. V. & Keiser, M. J. Adversarial controls for scientific machine learning. ACS Chem. Biol.13, 2819–2821 (2018). CASPubMedGoogle Scholar
- Beker, W., Gajewska, E. P., Badowski, T. & Grzybowski, B. A. Prediction of major regio-, site-, and diastereoisomers in diels-alder reactions by using machine-learning: the importance of physically meaningful descriptors. Angew. Chem. Int. Ed.58, 4515–4519 (2019). CASGoogle Scholar
- Nielsen, M. K., Ahneman, D. T., Riera, O. & Doyle, A. G. Deoxyfluorination with sulfonyl fluorides: navigating reaction space with machine learning. J. Am. Chem. Soc.140, 5004–5008 (2018). CASPubMedGoogle Scholar
- Halford, G. S., Baker, R., McCredden, J. E. & Bain, J. D. How many variables can humans process? Psychol. Sci.16, 70–76 (2005). PubMedGoogle Scholar
- Leardi, R. Experimental design in chemistry: A tutorial. Anal. Chim. Acta652, 161–172 (2009). CASPubMedGoogle Scholar
- Murray, P. M. et al. The application of design of experiments (DoE) reaction optimisation and solvent selection in the development of new synthetic chemistry. Org. Biomol. Chem.14, 2373–2384 (2016). CASPubMedGoogle Scholar
- Austin, N. D., Sahinidis, N. V., Konstantinov, I. A. & Trahan, D. W. COSMO-based computer-aided molecular/mixture design: A focus on reaction solvents. AIChE J.63, 104–122 (2018). Google Scholar
- Struebing, H. et al. Computer-aided molecular design of solvents for accelerated reaction kinetics. Nat. Chem.5, 952–957 (2013). CASPubMedGoogle Scholar
- Truhlar, D. G. Chemical reactivity: Inverse solvent design. Nat. Chem.5, 902–903 (2013). CASPubMedGoogle Scholar
- Gao, H. et al. Using machine learning to predict suitable conditions for organic reactions. ACS Cent. Sci.4, 1465–1476 (2018). CASPubMedPubMed CentralGoogle Scholar
- Zhou, Z., Li, X. & Zare, R. N. Optimizing chemical reactions with deep reinforcement learning. ACS Cent. Sci.3, 1337–1344 (2017). CASPubMedPubMed CentralGoogle Scholar
- Bedard, A. C. et al. Reconfigurable system for automated optimization of diverse chemical reactions. Science361, 1220–1225 (2018). CASPubMedGoogle Scholar
- Reker, D. & Schneider, G. Active-learning strategies in computer-assisted drug discovery. Drug. Discov. Today20, 458–465 (2015). PubMedGoogle Scholar
- Reker, D., Schneider, P. & Schneider, G. Multi-objective active machine learning rapidly improves structure–activity models and reveals new protein–protein interaction inhibitors. Chem. Sci.7, 3919–3927 (2016). CASPubMedPubMed CentralGoogle Scholar
- Reker, D. & Brown, J. B. Selection of informative examples in chemogenomic datasets. Methods Mol. Biol.1825, 369–410 (2018). CASPubMedGoogle Scholar
- Reker, D., Schneider, P., Schneider, G. & Brown, J. B. Active learning for computational chemogenomics. Future Med. Chem.9, 381–402 (2017). CASPubMedGoogle Scholar
- Sans, V., Porwol, L., Dragone, V. & Cronin, L. A self optimizing synthetic organic reactor system using real-time in-line NMR spectroscopy. Chem. Sci.6, 1258–1264 (2015). CASPubMedGoogle Scholar
- Häse, F., Roch, L. M., Kreisbeck, C. & Aspuru-Guzik, A. Phoenics: A Bayesian optimizer for chemistry. ACS Cent. Sci.4, 1134–1145 (2018). PubMedPubMed CentralGoogle Scholar
- Frazier, P. I. A tutorial on Bayesian optimization. Preprint at arXivhttps://arxiv.org/abs/1807.02811 (2018).
- Brochu, E., Cora, V. M. & Freitas, N. d. A tutorial on Bayesian optimization of expensive cost functions, with application to active user modeling and hierarchical reinforcement learning. Preprint at arXivhttps://arxiv.org/abs/1012.2599 (2010).
- Reker, D., Bernardes, G. J. L. & Rodrigues, T. Evolving and nano data enabled machine intelligence for chemical reaction optimization. Preprint at ChemRxivhttps://chemrxiv.org/articles/Evolving_and_Nano_Data_Enabled_Machine_Intelligence_for_Chemical_Reaction_Optimization/7291205/1 (2018).
- Granda, J. M., Donina, L., Dragone, V., Long, D. L. & Cronin, L. Controlling an organic synthesis robot with machine learning to search for new reactivity. Nature559, 377–381 (2018). CASPubMedPubMed CentralGoogle Scholar
- Ahmadi, M., Vogt, M., Iyer, P., Bajorath, J. & Frohlich, H. Predicting potent compounds via model-based global optimization. J. Chem. Inf. Model.53, 553–559 (2013). CASPubMedGoogle Scholar
- Patil, P. C. & Luzzio, F. A. Synthesis of extended oxazoles II: Reaction manifold of 2-(halomethyl)-4,5-diaryloxazoles. Tetrahedron Lett.57, 757–759 (2016). CASPubMedPubMed CentralGoogle Scholar
- Blakemore, D. C. et al. Organic synthesis provides opportunities to transform drug discovery. Nat. Chem.10, 383–394 (2018). CASPubMedGoogle Scholar
- Roberts, R. M. Serendipity: Accidental Discoveries in Science 1-288 (John Wiley & Sons, 1989).
- Davey, S. Rapid reaction discovery. Nat. Chem.4, 69 (2012). CASGoogle Scholar
- McNally, A., Prier, C. K. & MacMillan, D. W. Discovery of an alpha-amino C–H arylation reaction using the strategy of accelerated serendipity. Science334, 1114–1117 (2011). CASPubMedPubMed CentralGoogle Scholar
- Amara, Z. et al. Automated serendipity with self-optimizing continuous-flow reactors. Eur. J. Org. Chem.2015, 6141–6145 (2015). CASGoogle Scholar
- Dragone, V., Sans, V., Henson, A. B., Granda, J. M. & Cronin, L. An autonomous organic reaction search engine for chemical reactivity. Nat. Commun.8, 15733 (2017). PubMedPubMed CentralGoogle Scholar
- Gromski, P. S., Henson, A. B., Granda, J. M. & Cronin, L. How to explore chemical space using algorithms and automation. Nat. Rev. Chem.3, 119–128 (2019). Google Scholar
- Cao, Y., Romero, J. & Aspuru-Guzik, A. Potential of quantum computing for drug discovery. IBM J. Res. Dev.62, 6:1–6:20 (2019). Google Scholar
- Rodrigues, T. et al. Multidimensional de novo design reveals 5-HT2B2B receptor-selective ligands. Angew. Chem. Int. Ed.54, 1551–1555 (2015). CASGoogle Scholar
- Reutlinger, M., Rodrigues, T., Schneider, P. & Schneider, G. Combining on-chip synthesis of a focused combinatorial library with computational target prediction reveals imidazopyridine GPCR ligands. Angew. Chem. Int. Ed.53, 582–585 (2014). CASGoogle Scholar
- Ban, T. A. The role of serendipity in drug discovery. Dialogues Clin. Neurosci.8, 335–344 (2006). PubMedPubMed CentralGoogle Scholar
- Rosales, A. R. et al. Rapid virtual screening of enantioselective catalysts using CatVS. Nat. Catal.2, 41–45 (2019). CASGoogle Scholar
- Steiner, S. et al. Organic synthesis in a modular robotic system driven by a chemical programming language. Science363, eaav2211 (2019). CASPubMedGoogle Scholar
- Caramelli, D. et al. Networking chemical robots for reaction multitasking. Nat. Commun.9, 3406 (2018). PubMedPubMed CentralGoogle Scholar
- Fitzpatrick, D. E., Maujean, T., Evans, A. C. & Ley, S. V. Across-the-world automated optimization and continuous-flow synthesis of pharmaceutical agents operating through a cloud-based server. Angew. Chem. Int. Ed.57, 15128–15132 (2018). CASGoogle Scholar
- Lavecchia, A. Machine-learning approaches in drug discovery: methods and applications. Drug Discov. Today20, 318–331 (2015). PubMedGoogle Scholar
- Butler, K. T., Davies, D. W., Cartwright, H., Isayev, O. & Walsh, A. Machine learning for molecular and materials science. Nature559, 547–555 (2018). CASPubMedGoogle Scholar
- Jordan, M. I. & Mitchell, T. M. Machine learning: Trends, perspectives, and prospects. Science349, 255–260 (2015). CASPubMedGoogle Scholar
- Sanchez-Lengeling, B. & Aspuru-Guzik, A. Inverse molecular design using machine learning: Generative models for matter engineering. Science361, 360–365 (2018). CASPubMedGoogle Scholar
- Wallach, I. & Heifets, A. Most ligand-based classification benchmarks reward memorization rather than generalization. J. Chem. Inf. Model.58, 916–932 (2018). CASPubMedGoogle Scholar
Acknowledgements
A.F.A. acknowledges Fundação para a Ciência e Tecnologia (FCT) Portugal for financial support through a PhD grant (PD/BD/143125/2019). T.R. is an investigador auxiliar supported by FCT Portugal (CEECIND/00887/2017). T.R. acknowledges FCT/FEDER (02/SAICT/2017, grant 28333) for funding. The authors thank the reviewers for their comments.